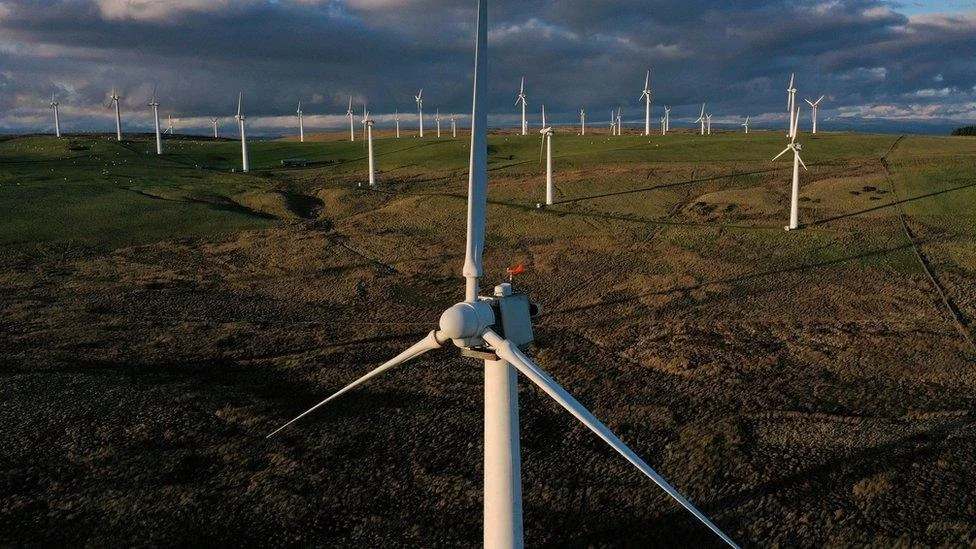
Wind power resources for Northeast US under a changing climate
Lead PI: Dr. Marina Astitha, UAlbany
Co-PI: Dr. Jeff Freedman, UConn
Background:
This proposal focuses on how climate change will affect wind energy resources, both onshore and offshore, in the Northeastern United States. Although many states have ambitious targets for transitioning to 100% renewable energy by mid-century, the variability and future distribution of wind speed—especially at finer spatial scales—remain sources of significant uncertainty. Previous research suggests that current general circulation models do not adequately capture localized wind behaviors, making downscaling techniques critical for accurately estimating wind resource availability.
Industry Need:
As wind becomes a key component in the clean energy mix, power producers, utilities, and financiers require detailed assessments that capture not only mean wind speeds but also the probability of extreme conditions. Events such as “wind droughts,” where capacity factors dip below 50% for sustained periods, can substantially affect project economics and reliability. Moreover, the siting, design, and financing of wind farms depend heavily on accurate wind data and projections to minimize financial and operational risks over the multi-decade operational horizon of wind energy projects
Objectives:
The project aims to quantify how wind speeds at both near-surface and hub-height levels might change under future climate scenarios, focusing on (1) changes in average wind patterns and associated uncertainty, (2) potential impacts to the energy production of specific onshore and offshore wind farms, and (3) the likelihood of extreme events that significantly diminish wind power generation. By delivering robust probabilistic data on wind variability and extremes, the research helps stakeholders prepare for the long-term implications of climate change.
Methodology:
Two complementary downscaling techniques—statistical and dynamic—will be employed to refine coarse global climate model outputs to resolutions as fine as 4–6 km and time steps as detailed as hourly or three-hourly. Statistical downscaling applies regression and analog methods to predict future wind speeds based on historical observational data, whereas dynamic downscaling uses the Weather Research and Forecasting (WRF) model for a physics-based approach to regional climate projections. By comparing outputs from multiple global climate models under two emissions scenarios (SSP2-4.5 and SSP5-8.5), the project will provide a more comprehensive view of uncertainty and variability, including factors such as diurnal wind patterns and extreme weather events.
Deliverables:
The researchers will produce high-resolution datasets that span the onshore and offshore regions of the Northeastern United States. These datasets—covering the period from 2024 to 2070—will include maps of projected wind speed changes, estimates of wind capacity factor shifts, and assessments of the probability of extreme wind events like wind droughts. The final analysis will feature uncertainty bounds to help industry stakeholders better evaluate financial and operational risks, plan for future development, and integrate these insights into broader energy system resiliency measures.