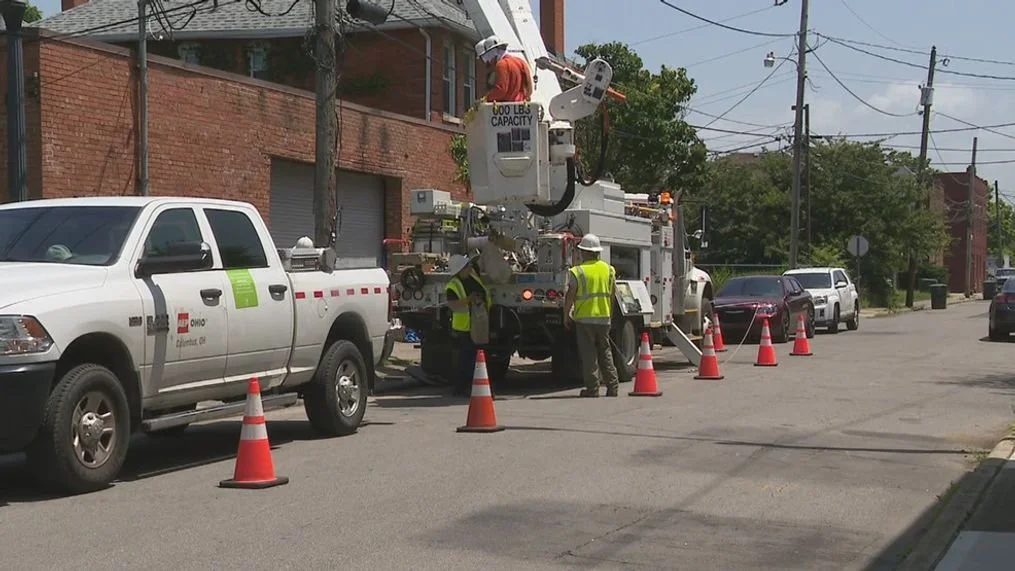
Integrating Interdisciplinary Resilience Indices for Power Outages and Restorations
Lead PI: Dr. Wei Zhang, UConn
Co-PI: Ross Bagtzoglou, UConn
Background:
The document highlights the growing need to enhance power system resilience in the face of adverse weather events, which can cause widespread power outages and long restoration times. Existing research often focuses on predicting the location or count of outages, but less attention is paid to integrating other infrastructure systems—such as transportation networks or tree-removal services—that can significantly influence outage duration. As economic losses from power outages increase with time, there is a demand for more holistic, interdisciplinary models to capture how multiple factors affect both damage extent and restoration timelines.
Industry Need:
Because standard industry metrics (e.g., SAIDI and SAIFI) primarily track the frequency and duration of interruptions, utility and infrastructure planners require a more robust assessment of how different infrastructure components interact in real-world scenarios. For instance, blocked roads, fallen trees, and limited crew resources can all increase the difficulty of restoring electricity service. Integrating these factors in outage prediction and restoration models offers electric utilities better insights into budgeting for grid-hardening strategies, managing resources, and planning for extreme weather events under future climate scenarios.
Objectives:
The project aims to identify and incorporate previously neglected parameters—ranging from tree-management policies to transportation infrastructure resilience—so that SAIDI and SAIFI calculations reflect a truly holistic perspective. By examining how power and transportation systems interact during extreme weather events, it seeks to improve both outage prediction and restoration modeling. An additional objective is to use these enhanced models to inform decisions on grid-hardening measures, such as improved vegetation management, structural upgrades, or expanded repair crews, and to evaluate the cost-effectiveness of different resilience options under varying climate and budget scenarios.
Methodology:
A post-storm restoration Total System Model (PRTSM) will unify existing outage prediction tools, pole-wire structural fragility assessments, economic analyses, and agent-based simulation of repair crews. From the top down, the model incorporates wide-ranging resilience indicators—such as storm intensity and potential tree falls—while an agent-based component captures the bottom-up perspective of how crews travel across disrupted roads to restore service. This integrated modeling approach refines estimates of restoration times by computing probabilities of road blockages, analyzing crew movements in real time, and exploring how varying resource distributions affect overall system recovery.
Deliverables:
Key outcomes include a validated tool calibrated against historical storm data, enabling probabilistic analyses of outage and restoration scenarios that align with annual SAIDI and SAIFI metrics. By simulating diverse “what-if” conditions—ranging from climate-induced storm changes to shifts in crew allocation strategies—this approach will guide utilities and policymakers in prioritizing and justifying resilience investments. The work also lays the groundwork for future interdisciplinary research supported by entities like NSF, DOE, NOAA, and FEMA, strengthening the link between power system resilience and broader infrastructure considerations.