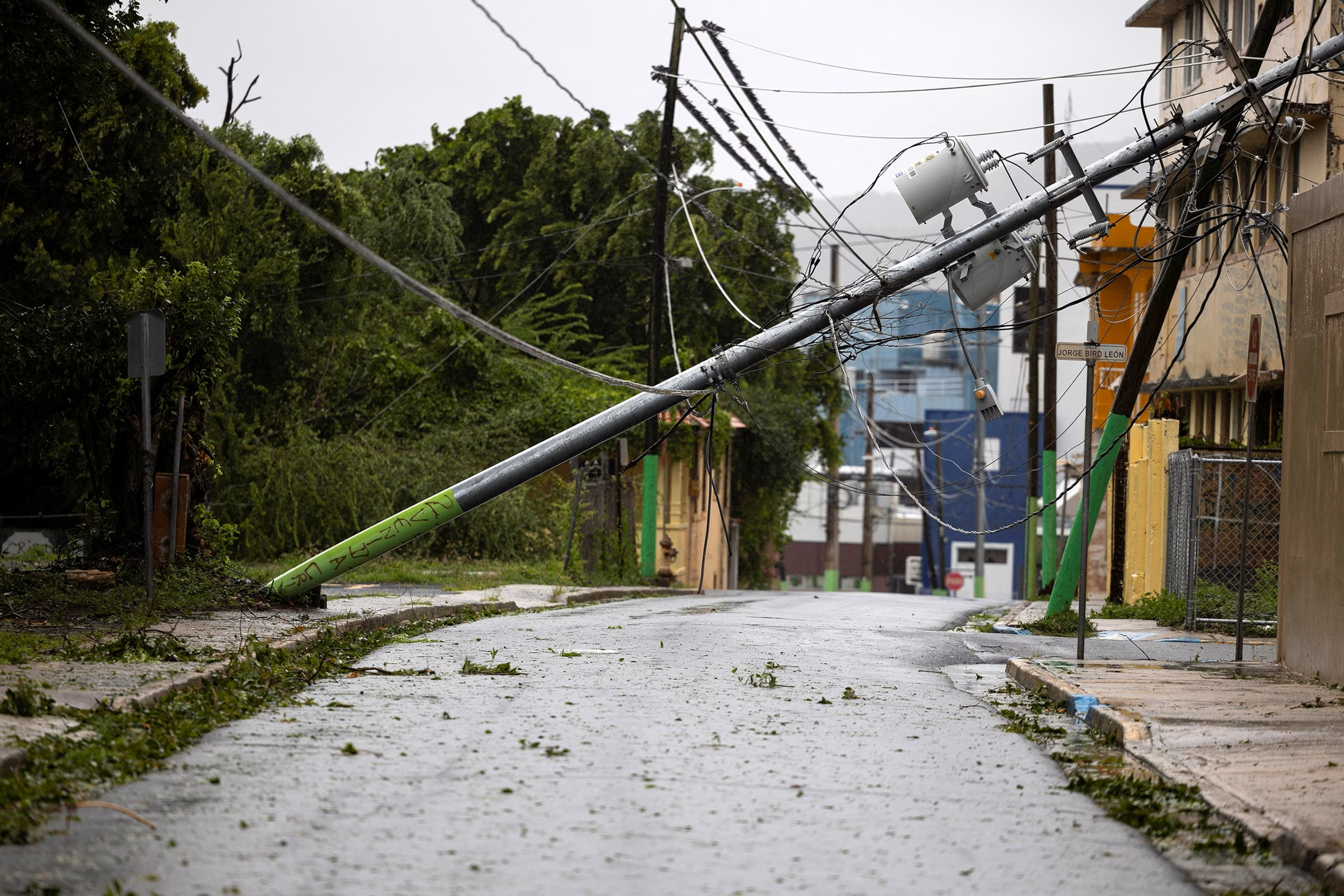
Predicting Extreme Weather-Induced Power Outages with Spatially-Aware Hybrid Graph Neural Networks
Lead PI: Dr. Dongjin Song, UConn
Background:
Extreme weather events like hurricanes, storms, and ice storms, exacerbated by climate change, frequently cause widespread power outages. These disruptions impact industries, communities, and critical infrastructure, affecting economic stability and public services. The University of Connecticut developed the Outage Prediction Modeling (OPM) system to forecast power outages before such events occur. However, while OPM performs well for low-to-medium-impact weather conditions, its accuracy in extreme weather scenarios remains inadequate. Furthermore, existing models, such as Random Forest and XGBoost, do not consider spatial relationships among affected areas. This project aims to improve outage prediction by developing Spatially-Aware Hybrid Graph Neural Networks (SA-HGNNs) that incorporate both spatial and dynamic factors.
Industry Need:
The improved OPM system is expected to transform how utility companies prepare for and respond to extreme weather risks. The study aims to enhance OPM’s predictive accuracy for extreme weather-induced outages by analyzing spatial effects. Also provide utility companies with improved outage management strategies to optimize resource allocation, reduce downtime, and improve service reliability.
Objectives:
The project aims to develop SA-HGNNs to predict power outages by integrating static (land cover, infrastructure) and dynamic (wind speed, precipitation) features. Address the data imbalance problem related to different types of extreme weather events and improve prediction accuracy by incorporating spatial location biases and comparing SA-HGNNs with existing models
Deliverables:
The expected outputs include a fully developed SA-HGNNs framework for power outage prediction. Also, a comparative analysis report detailing the performance of SA-HGNNs against existing models across different extreme weather scenarios and a set of recommendations for utility companies on implementing SA-HGNNs for outage management and emergency planning.