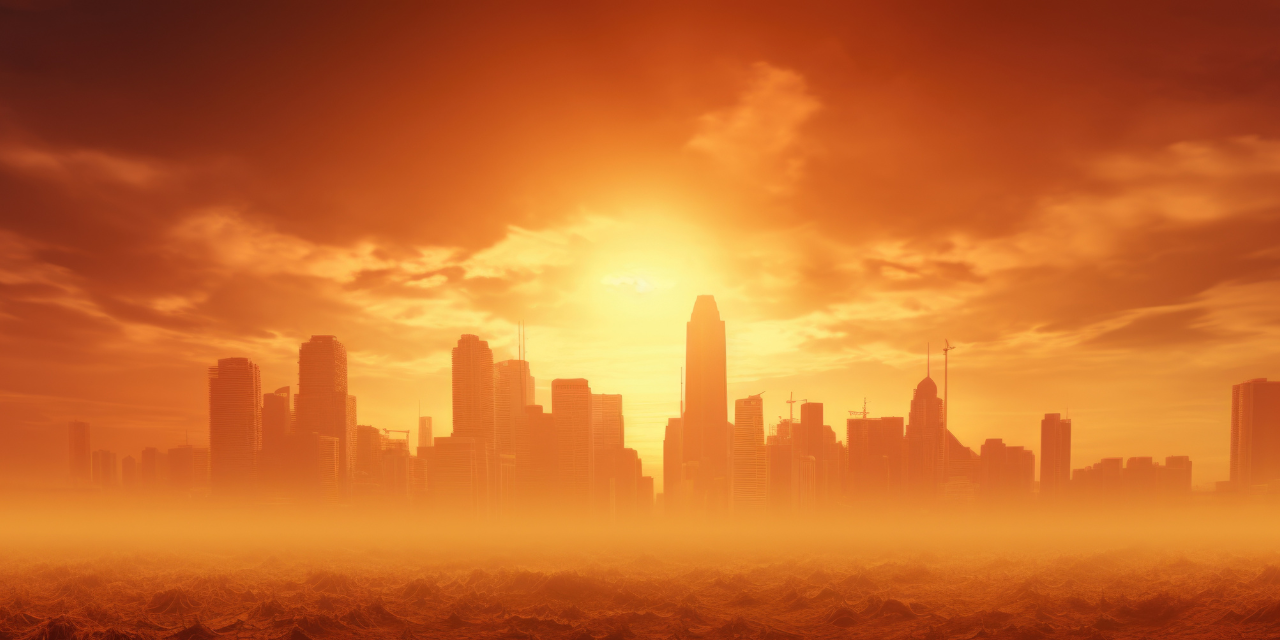
Extreme Heat Metrics for More Accurate Energy Demand Prediction
Lead PI: Dr. Guiling Wang, UConn
Co-PI: Dr. Yara Medawar, UConn
Background:
Extreme heat events are causing significant increases in electricity demand due to rising air conditioning usage, posing risks to power grid stability. Traditional energy demand forecasting relies on maximum or average temperature as a key predictor, but wet bulb temperature, which accounts for humidity, more accurately represents human thermal comfort and may better predict energy consumption patterns. A more precise energy demand prediction model incorporating wet bulb temperature could improve grid resilience, enhance load management, and stabilize electricity markets.
Industry Need:
Accurate energy demand forecasting during extreme heat events is critical for maintaining grid reliability and preventing disruptions such as rolling power outages. Current models often fail to capture the full impact of heat events, leading to inefficiencies in power distribution and market volatility. The energy sector requires improved predictive tools that integrate multiple heat metrics to enhance operational planning, optimize resource allocation, and ensure grid stability.
Objectives:
This project aims to improve energy demand prediction during extreme heat events by incorporating wet bulb temperature into forecasting models. Key objectives include analyzing the diurnal cycles of dry and wet bulb temperatures, comparing them with energy consumption patterns, and examining day-to-day variability in demand based on different heat metrics. The goal is to develop a more accurate model for predicting daily and peak electricity demand at state and regional levels.
Methodology:
The study will use historical electricity demand data from ISO-New England and NYISO, along with meteorological data from NOAA’s Global Historical Climatology Network. Wet bulb temperature will be calculated using established empirical methods and analyzed alongside traditional heat metrics. Relationships between energy demand and temperature variables will be assessed across different timescales, with a focus on extreme heat events. The project will also explore how wet bulb temperature influences the timing of peak energy demand, providing insights into potential phase shifts in electricity consumption.
Deliverables:
The project will produce two prototype models: one predicting daily electricity demand and another forecasting peak demand and its timing, both based on heat metrics. The findings will improve energy market stability and grid resilience, helping utilities and policymakers better anticipate and manage electricity demand during heatwaves. The results will contribute to a more reliable power grid and informed decision-making in the face of climate variability and extreme weather events.