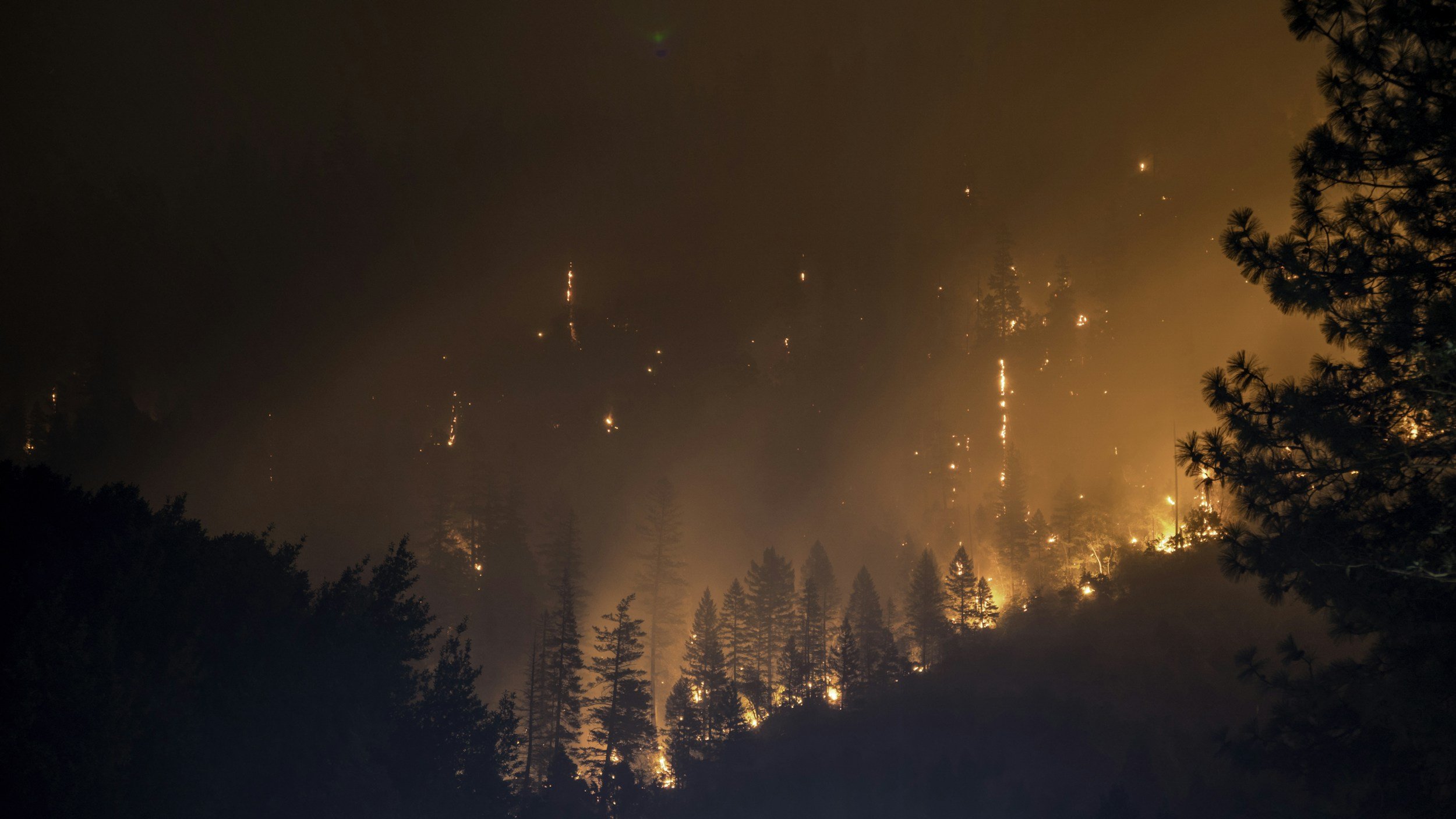
Modeling Fire Weather in New England
Lead PI: Dr. Robert Fovell, UAlbany
Co-PI: Dr. Diego Cerrai, UConn; Dr. Scott Capps (Technosylva)
Background:
In the U.S., about 67,000 wildfires burn 7 million acres annually. While most are extinguished quickly, some persist to devastate large areas, such as the November 2018 Camp Fire in California. This was the year’s most expensive global natural disaster and contributed to Pacific Gas and Electric’s (PG&E) bankruptcy. Concern regarding utility-caused fires in California has led to the implementation of proactive Public Safety Power Shutoffs (PSPS) during threatening conditions. Compared to the western U.S., however, there is a gap in our knowledge and understanding of wildfires in the northeast U.S.. Fire occurrence here is not uncommon, especially in March-May, with the majority of ignitions having human and infrastructure causes, including debris burning and power line faults. We propose to create a 30-year historical database of important measures, including wind, humidity, and precipitation. This will be used to compute fire weather related products such as dead fuel moisture (DFM), drought indices, and energy release component (ERC). We will also create an index that, together with the historical weather information, will be used as input to the UConn Wildfire Ignition Model (WIM), a machine-learning based system under development at UConn. Once fully developed, this system will allow federal and state governments, utilities, and other stakeholders to know in advance the location of possible fires and take actions for mitigating their impact or preventing their onset.
Objectives:
To predict the future, we need to understand the past. We propose to create a 30-year historical database of important measures, including wind, humidity, and precipitation. This will be used to compute fire weather related products such as dead fuel moisture (DFM), drought indices, and energy release component (ERC). We will also create an index that, together with the historical weather information, will be used as input to the UConn Wildfire
Ignition Model (WIM), a machine-learning based system under development at UConn. Once fully developed, this system will allow federal and state governments, utilities, and other stakeholders to know in advance the location of possible fires and take actions for mitigating their impact or preventing their onset.
Deliverables:
A 30-year climatology of weather, fuels, and fire potential index will be developed, putting current and future events into historical context. The index can be computed from current operational forecast model outputs for enhanced situational awareness. The newly generated environmental information will allow for the validation of the WIM over an area (the northeast U.S.) different than the one where it was originally developed (California).