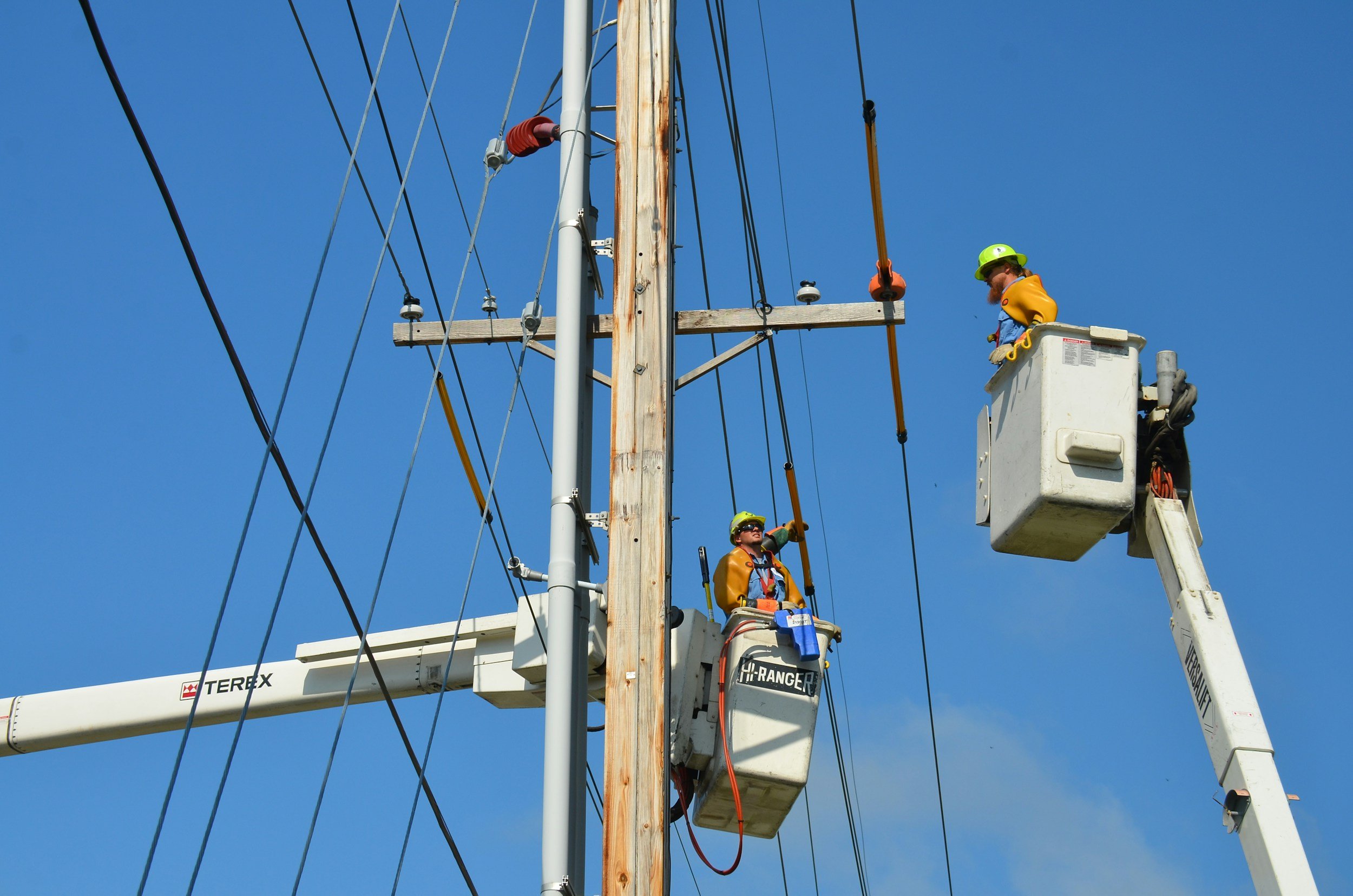
Prediction Uncertainty in Power Outages Connected to Weather Forecast Lead-Time
Lead PI: Dr. Xinxuan Zhang, UConn
Co-PI: Dr. Marina Astitha, UConn
Background:
The UConn Outage Prediction Modeling (OPM) system has been in real-time operation for a decade, offering pre-storm power outage forecasts in electric distribution systems. The machine learning based OPM is trained offline with historical weather data, then applied in forecast mode using weather forecast products for upcoming storms. While the performance of historical mode OPM has undergone comprehensive evaluation during the model development phase, there remains a lack of understanding in the forecast mode OPM predictability limits introduced by the uncertainty of weather forecasts. Given the OPM system is recently extending the forecast lead times from 3 days ahead of a storm to 5 days ahead, the need for quantitative analysis on the OPM forecast uncertainty becomes more necessary.
Industry Relevance:
1. This study will further interpret the forecast mode OPM outputs by considering model uncertainties with different weather forecast lead times. Understanding the quality of longer lead-time forecasts will particularly be useful to support utility preparedness for major storms.
2. The trends of OPM forecast skill may vary for different storm types (i.e. rain and wind events, thunderstorms, snow and ice events). These results will guide the future direction of the OPM continuous development plan.
Objectives:
1. Evaluate the uncertainty of weather variables with different forecast lead-times for different storm types.
2. Evaluate the skill of the outage prediction model from different weather forecast lead-times for different storm types.
3. Assess the error propagated from weather forecasts to outage predictions.